How Uber Solved the Asymmetric Supply Problem
- Sambeet Parija
- Mar 7
- 4 min read
This problem of asymmetric, unpredictable supply is fundamental to any gig economy platform that relies on freelance workers. Solving it requires nudging behavior without forcing it, since these aren’t employees.
How Uber Used Nudges, Signals, and Smart Incentives to Solve the Peak-Hour Problem
If you’ve ever opened the Uber app during rush hour and seen longer wait times or higher prices, you’ve experienced one of the platform’s most critical early challenges: fluctuating driver availability during peak demand. This wasn’t just an occasional hiccup, it posed a serious risk to Uber’s promise of fast, reliable rides. Here's how Uber engineered a scalable and elegant solution using a mix of behavioral economics, technology, and data science.
Rider demand on Uber tends to spike during specific high-traffic periods, particularly during morning and evening commute hours when people are traveling to and from work. Demand also rises significantly on weekends, during festivals, and at times of large public events like concerts or sports games. Additionally, bad weather conditions often lead to a surge in ride requests, as people avoid walking or using public transport, further increasing pressure on driver availability.

But most Uber drivers are independent contractors, which means they have no fixed schedules and are under no obligation to work during peak hours. This flexibility, while attractive to drivers, often leads to a mismatch during high-demand periods, as many choose to stay offline or avoid traffic-heavy or congested areas, further aggravating the supply-demand imbalance.
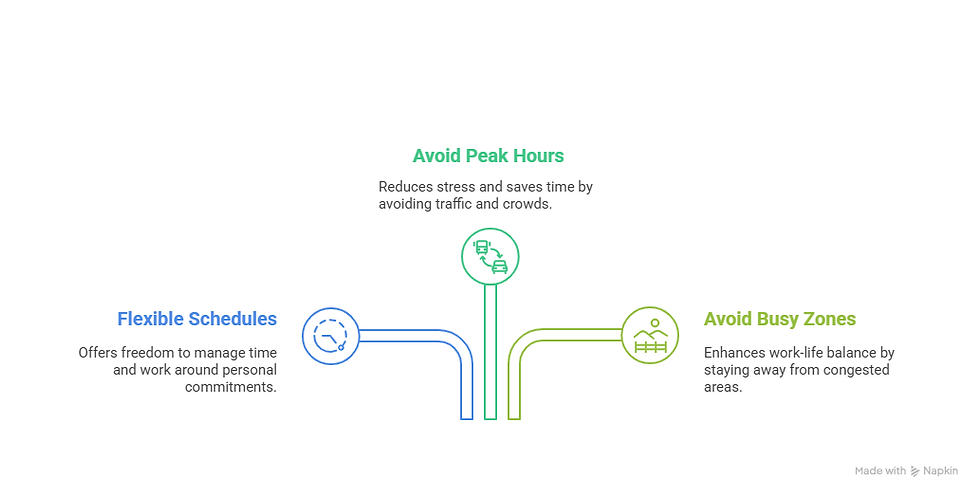
This mismatch between rider demand and driver availability led to a cascade of negative outcomes. Riders experienced increased wait times and a higher likelihood of cancellations, especially during peak periods. The introduction of surge pricing, while effective in incentivizing drivers, often triggered backlash from users who perceived it as unfair. Over time, these issues contributed to a gradual loss of user trust, threatening Uber’s reputation for reliability and convenience.
Uber's solution was multi-pronged and one of the best examples of how to address asymmetric supply in a gig economy.

Surge Pricing (Dynamic Pricing)
When rider demand exceeds the number of available drivers in a particular area, Uber activates surge pricing. This feature temporarily increases fares in high-demand zones, effectively attracting more drivers to those areas. The higher payouts provide a strong financial incentive for drivers to log in and move toward surge zones. At the same time, surge pricing helps moderate demand by encouraging only riders with urgent needs to book, thereby rebalancing the system more efficiently.
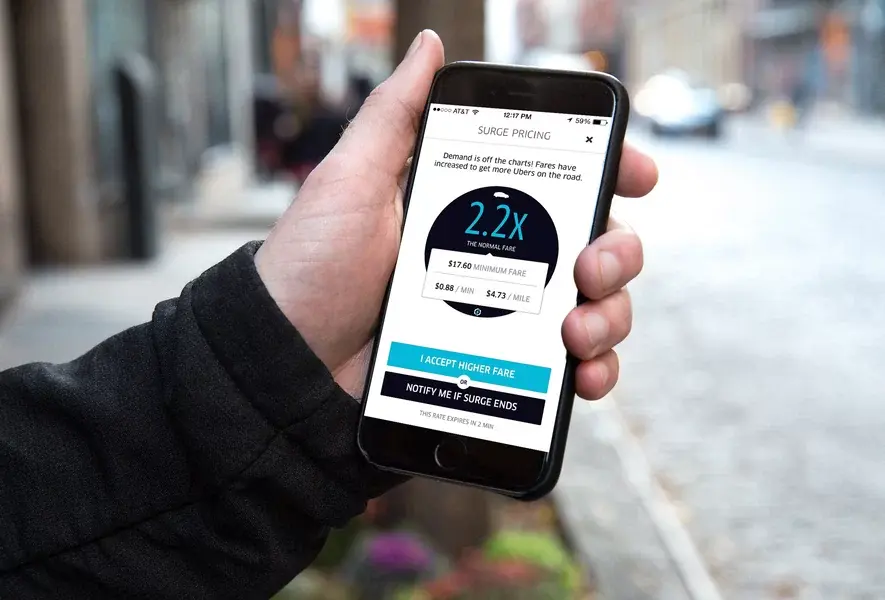
Driver Incentives and Earnings Guarantees
To further ensure driver availability during peak hours, Uber introduced a range of performance-based incentives. One popular initiative is the “Quest” bonus, which offers drivers a cash reward for completing a specific number of trips within a designated timeframe. For instance, completing 50 trips between certain time frame might earn a driver an additional $145. In some regions, Uber also provides hourly earnings guarantees, ensuring drivers are compensated fairly even during short lulls between trips. These incentives are designed to make peak-time driving more appealing and predictable.

Real-Time Heatmap
Uber equips its drivers with a real-time heatmap feature that displays areas of high rider demand. These zones are visually highlighted within the driver app, helping drivers quickly identify where they are most likely to get ride requests. By guiding drivers toward high-earning areas, this feature not only improves driver efficiency and income but also helps ensure better rider coverage in busy locations.

Predictive Analytics and Notifications
Behind the scenes, Uber’s platform uses machine learning models to analyze historical patterns, current traffic, weather data, and event schedules to forecast demand surges before they happen. Based on these insights, Uber proactively sends push notifications to drivers, alerting them of expected demand in specific areas. These timely nudges encourage drivers to get online ahead of the surge, positioning supply right where and when it’s needed most.
Expanding the Flexible Driver Pool
To address fluctuating demand with a resilient supply base, Uber has invested heavily in expanding its pool of flexible drivers. The platform streamlines the onboarding process to minimize friction, making it easy for new drivers to join. Additionally, Uber’s driver app allows existing drivers to log in, go offline, or relocate with minimal effort, offering maximum flexibility. This adaptability is crucial in ensuring that supply can quickly respond to demand fluctuations, especially during unpredictable peak periods.
Final Thoughts
The challenge of driver availability during peak hours isn’t unique to Uber, it’s a common issue faced by all gig economy platforms that rely on flexible, independent workers. What sets Uber apart is how it solved this problem without enforcing control, instead using a thoughtful combination of nudges, signals, and well-designed incentives to influence behavior at scale.
This approach became a blueprint for the industry. Companies like DoorDash, Instacart, and others have since adopted similar strategies to manage supply demand imbalances in their own ecosystems. Uber’s ability to align the actions of millions of independent contractors through data driven, behaviorally informed design remains one of its most powerful innovations.
We’d love to hear your thoughts. Have you seen similar strategies used in other platforms or industries? Drop your insights in the comments below and share this post if it sparked any ideas.
Comentarios